12.04 MOnitoring, MOdeling and forecasting MObility patterns (MO3)
While an established mobility modelling paradigm, based on limited data, has served us well during the past decades, currently emerging technologies provide an opportunity for a disruptive paradigm shift. Leveraging a wealth of ubiquitous, heterogeneous data that is rapidly becoming available, in conjunction with state-of-the-art modelling techniques, it is possible to model human mobility patterns at an unprecedented level of accuracy and fidelity, possibly in real-time. Within this project, the TUM chairs of Transportation Systems Engineering (TSE) and Connected Mobility (CM) will join their complementary and interdisciplinary expertise to achieve the ambitious goals set out. In particular, we will use the expertise of CM to devise measurement systems and query infrastructure and inference models, with the aim of developing recommender systems as a possible application. Furthermore, building on previous related TSE work, we will develop behavioural mobility models, integrate (observed/inferred) demand onto a demand/supply modeling environment, and use measurements to calibrate integrated models. We will leverage our network of international collaborators to obtain additional data sets and expertise, and benefit the participating researchers through international mobility research visits. The close collaboration of the interdisciplinary team is expected to result in a fruitful and long-lasting research relationship, which will lead to many other joint research activities, also following the successful completion of the MO3 project.
Update from the project team, a blurb from the latest research article (see publications):
A close collaboration between Gemeinde Kirchheim, a mobility innovation-facilitating city north-west of Munich, and the Chairs of Transportation Systems Engineering and Connected Mobility of TUM aims at moving traffic monitoring forward. Mobility behavior and patterns of user of all transportation modes are to be recorded by using existing transport infrastructure as hosts for communication, weather, and noise sensors. The accuracy of traffic modelling, planning, and estimation will be advanced by correlating and analyzing gathered and additional external heterogeneous data sources.
Journal papers:
M. Sadrani, A. Tirachini and C. Antoniou, (2021). Vehicle dispatching plan for minimizing passenger waiting time in a corridor with buses of different sizes: Model formulation and solution approaches, European Journal of Operational Research, doi.org/10.1016/j.ejor.2021.07.054
S. Su, E. Chaniotakis, S. Narayanan, H. Jiang and C. Antoniou (2020). Clustered tabu search optimization for reservation-based shared autonomous vehicles, Transportation Letters, DOI: 10.1080/19427867.2020.1824309
Tirachini, A., E. Chaniotakis, M. Abouelela and C. Antoniou (2020). The sustainability of shared mobility: Can a platform for shared rides reduce motorized traffic in cities? Transportation Research Part C 117 (2020) 102707
Timokhin, S.; Sadrani, M.; Antoniou, C. Predicting Venue Popularity Using Crowd-Sourced and Passive Sensor Data. Smart Cities 2020, 3, 818-841. (Open access: www.mdpi.com/2624-6511/3/3/42)
Qurashi, M., T. Ma, E. Chaniotakis and C. Antoniou (2019). PC-SPSA: Employing Dimensionality Reduction to Limit SPSA Search Noise in DTA Model Calibration, IEEE Transactions on Intelligent Transportation Systems, Volume: 21 , Issue: 4 , April 2020. DOI: 10.1109/TITS.2019.2915273
Duran-Rodas, D., E. Chaniotakis and C. Antoniou (2019). Built Environment Factors Affecting Bike Sharing Ridership: A Data-Driven Approach for Multiple Cities. Transportation Research Record: Journal of the Transportation Research Board.
Conference papers:
A. Fatola, T. Ma and C. Antoniou (2021). Modeling and Forecasting Individual On-Demand Upcoming Trips, the 7th International Conference on Models and Technologies for Intelligent Transportation Systems (MT-ITS), doi.org/10.1109/MT-ITS49943.2021.9529314
S. Y. Y. Hamad, T. Ma and C. Antoniou (2021). Analysis and Prediction of Bikesharing Traffic Flow – Citi Bike, New York, the 7th International Conference on Models and Technologies for Intelligent Transportation Systems (MT-ITS), doi.org/10.1109/MT-ITS49943.2021.9529290
X. Gong, T. Ma, and C. Antoniou (2021). Network Traffic Dynamics Prediction with a Hybrid Approach: Autoencoder-VAR, the 7th International Conference on Models and Technologies for Intelligent Transportation Systems (MT-ITS), doi.org/10.1109/MT-ITS49943.2021.9529299
Duran-Rodas, D., E. Chaniotakis and C. Antoniou (2019). Built Environment Factors Affecting Bike Sharing Ridership: A Data-Driven Approach for Multiple Cities. Proceedings of the 98th Annual Meeting of the Transportation Research Board, January 2019, Washington, D.C.
Tonetto, Leonardo, Moritz Untersperger, and Jörg Ott. "Towards Exploiting Wi-Fi Signals From Low Density Infrastructure for Crowd Estimation." Proceedings of the 14th Workshop on Challenged Networks. 2019.
Book chapters:
Durán-Rodas, D., E. Chaniotakis, G. Wulfhorst and C. Antoniou (2019). Open source data-driven method to identify most influencing spatiotemporal factors. An example of station-based bike sharing. In K. G. Goulias and A. W. Davis (eds), Mapping the Travel Behavior Genome, 1st Edition, 9780128173404.
Team
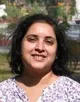
Project team leader
Dr. Santa Maiti
Chair of Transportation Systems Engineering
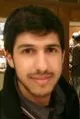
Doctoral researcher
Mohammad Sadrani
Chair of Transportation Systems Engineering
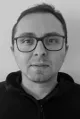
Doctoral Researcher
Leonardo Tonetto
Chair of Connected Mobility
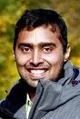
Doctoral researcher
Santhanakrishnan Narayanan
Chair of Transportation Systems Engineering
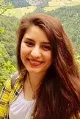
Doctoral Researcher
Pegah Torkamandi
Chair of Connected Mobiliy
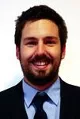
Alumnus
Emmanouil Chaniotakis
Chair of Transportation Systems Engineering
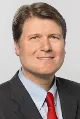
Principal Investigator
Professor Constantinos Antoniou
Chair of Transportation Systems Engineering
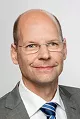
Principal Investigator
Professor Jörg Ott
Chair of Connected Mobility