AI-CHECK
Project: AI-enabled code compliance checking in early building design
Collaborating departments: Department of Civil and Environmental Engineering (TUM); Virtual Construction Lab (Technion).
Building Information Modelling (BIM) technologies have significantly improved building design efficiency. Nowadays, checking building models for compliance with building regulations by both the architect and the permitting authorities is performed at the certification stage. With the help of state-of-the-art BIM-based code compliance technologies, practitioners interpret the code compliance results and adjust the design manually based on their expertise. Many of these checks are conducted mostly manually and the process of design improvement is laborious and error-prone. To address those challenges, this project is to develop a methodology for automatically checking building designs against regulations, and then provide reasoning about any detected failures, which can assist in a systematic exploration of the design solution space for providing feasible design solutions.
In the research project AI-CHECK, we develop methods and techniques that support the automated checking and optimization of digital building models for compliance with construction codes. Providing applicable solutions involves optimizing the design space considering the distance to the currently failing solution. Developing such a methodology requires a formalization of the design space and the distance between models as an objective function. Additionally, the graph representation approach is employed to reveal the design intent and topology. Together, the graph representation of a set of models and their corresponding model-checking results will be used to design, train, and evaluate a deep-learning model that is capable of automatically verifying the compliance of new building models. Finally, as this project is tackling the early stages of design when checking a building design fails, we aim to develop methods that recommend suitable solutions by developing an AI-based optimization framework. We call this novel approach “code compliance healing”.
Bloch, T., Wu, T., Alnuzha, M. and Borrmann, A,2024. Leveraging graph based semantic enrichment for enhanced automated code-checking. In EC3 Conference 2024 (Vol. 4, pp. 0-0). European Council on Computing in Construction. MediaTUM
Wu, J., Esser, S., Nousias, S. and Borrmann, A., 2016. Enriching IFC Models with Spatial Design Logic and Parametrics to Improve Design Adaptability – the case of alignment grids. ICCCBE, 2024. MediaTUM
Wu, J., Nousias, S. and Borrmann, A., 2023. Parametrization-based solution space exploration for Model Healing. In Proc. of the 30th Int. Conference on Intelligent Computing in Engineering (EG-ICE). MediaTUM
Wu, J., Dubey, R.K., Abualdenien, J. and Borrmann, A., 2023. Model Healing: Toward a framework for building designs to achieve code compliance. In ECPPM 2022-eWork and eBusiness in Architecture, Engineering and Construction 2022 (pp. 450-457). CRC Press. MediaTUM
Team
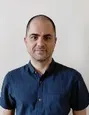
Coordinating Postdoc
Dr. Stavros Nousias
Chair of Computational Modeling and Simulation | TUM
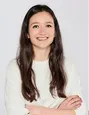
Doctoral Candidate
Fiona Claire Collins
Chair of Computational Modeling and Simulation | TUM
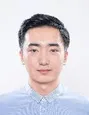
Doctoral Candidate
Wu, Jiabin
Chair of Computational Modeling and Simulation | TUM
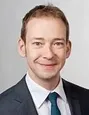
Principal Investigator
Prof. Dr. Ing. André Borrmann
Chair of Computational Modeling and Simulation | TUM
Principal Investigator
Prof. Rafael Sacks
Department of Electrical and Photonics Engineering | Technion