N-DAMN
Project: Next Generation Digital Acquisition using Modulo Nonlinearities: Algorithmic, Statistical and Information Theoretic Aspects
Collaborating Departments: Department of Electrical and Electronic Engineering (Imperial); Applied and Numerical Analysis and Optimization and Data Analysis (TUM)
By leveraging a co-design of mathematically guaranteed reconstruction algorithms and novel hardware, recent joint works by the PIs of this proposal put forth the Unlimited Sensing Framework (USF), a radically different approach for digitizing information. This approach overcomes the fundamental bottleneck of dynamic range (DR) that commonly arises when physical measurements overwhelm a sensor. More precisely, whenever a physical quantity (e.g. voltage, light flux, sound pressure) takes values beyond the DR of the sensor, the resulting measurements undergo a permanent information loss in the form of clipping or saturation. Instead of recording pointwise measurements (as is conventionally done), this approach is based on observing modulo measurements which fold out-of-range values into the dynamic range of the sensor. There on, by solving the inverse problem of recovering information from folded measurements, one recovers the high-dynamic-range (HDR) signal. So far, most of the investigation has been focused towards one-dimensional signals which rules out important applications such as imaging. Also to date, only limited mathematical analysis of the degree of information loss arising due to statistical noise is available. In this proposal, we address these challenges developing and analyzing efficient and robust reconstruction algorithms for multi-dimensional HDR acquisition via modulo architectures. To show that our mathematical insights can inspire new and innovative sensing pipelines, we will benchmark our algorithms with real data generated by our hardware prototype.
The focus of our project in the initial year has been on the statistical aspects of reconstruction from modulo samples. In the previous works between my research group and the group of Prof. Ayush Bhandari at Imperial College, we have only been able to treat bounded noise so far. Statistical noise, however, is in general unbounded. This can lead to spurious jumps in the reconstruction. Our strategy is to identify and remove these jumps using the theory of compressed sensing. This can be successful for moderate variances, as the jump vector is sparse. Consequently, we aim to recover using compressed sensing techniques such as ℓ1-minimization.
We worked on the stability and sensibility analysis of the original algorithm for the USF from the first paper ([BKR2017]) for unbounded additive statistical noise. A natural next step will be to study the distribution of estimates from the proofs in the paper, but since it is a challenging theoretical problem, we have been approaching it numerically. Parallel to the robustness of the algorithms and estimates in [BKR2017], we leveraged on the frequency-domain approach for the USF due to [BKP2021] to propose a novel procedure based on sparse recovery via ℓ1-minization, which is canonical in the Compressed Sensing community. That is an ongoing project whose numerical results look promising, but we still lack theoretical guarantees that would fit the so far nearly unexplored USF with unbounded statistical noise. Currently, we intend to achieve such guarantees via adaptation of previous results from the Sparse Recovery literature based on the conditioning of the measurement matrix.
Florescu, Dorian/Krahmer, Felix/Bhandari, Ayush (2022). The Surprising Benefits of Hysteresis in Unlimited Sampling: Theory, Algorithms and Experiments. In: IEEE Transactions on Signal Processing vol. 70: 616 - 630; DOI: 10.1109/TSP.2022.3142507.
Fernández-Menduiña, Samuel/Krahmer, Felix/Leus, Geert/Bhandari, Ayush (2022). Computational Array Signal Processing via Modulo Non-Linearities. In: IEEE Transactions on Signal Processing vol. 70: 2168 - 2179; DOI: 10.1109/TSP.2021.3101437.
Bhandari, Ayush (2022). Back in the US-SR: Unlimited Sampling and Sparse Super-Resolution With Its Hardware Validation. In: IEEE Signal Processing Letters vol. 29: 1047 - 1051; DOI: 10.1109/LSP.2022.3161865.
Florescu, Dorian/Bhandari, Ayush (2022). Time Encoding via Unlimited Sampling: Theory, Algorithms and Hardware Validation. In: IEEE Transactions on Signal Processing vol. 70: 4912 - 4924; DOI: 10.1109/TSP.2022.3210748.
Beckmann, Matthias/Bhandari, Ayush/ Krahmer, Felix (2022). The Modulo Radon Transform: Theory, Algorithms, and Applications. In: SIAM Journal on Imaging Sciences vol. 15 (no. 2): 455-490; DOI: 10.1137/21M1424615.
Conferences:
- Florescu, Dorian/Krahmer, Felix/Bhandari, Ayush (2021). Unlimited Sampling with Hysteresis. At: 2021 55th Asilomar Conference on Signals, Systems, and Computers, Pacific Grove, CA, USA. DOI: 10.1109/IEEECONF53345.2021.9723306
- Feuillen, Thomas/Alaee-Kerahroodi, Mohammad/Bhandari, Ayush/M.R. Shankar, Bhavani/Ottersten, Björn (2022). Unlimited Sampling for FMCW Radars: A Proof of Concept. At: 2022 IEEE Radar Conference (RadarConf22), New York City, NY, USA. DOI: 10.1109/RadarConf2248738.2022.9764291
- Florescu, Dorian/Bhandari, Ayush (2022). Unlimited Sampling with Local Averages. At: ICASSP 2022 - 2022 IEEE International Conference on Acoustics, Speech and Signal Processing (ICASSP), Singapore, Singapore. DOI: 10.1109/ICASSP43922.2022.9747127
- Bhandari, Ayush (2022). Unlimited Sampling with Sparse Outliers: Experiments with Impulsive and Jump or Reset Noise. At: ICASSP 2022 - 2022 IEEE International Conference on Acoustics, Speech and Signal Processing (ICASSP), Singapore, Singapore. DOI: 10.1109/ICASSP43922.2022.9746982
- Florescu, Dorian/Bhandari, Ayush (2022). Modulo Event-Driven Sampling: System Identification and Hardware Experiments. At: ICASSP 2022 - 2022 IEEE International Conference on Acoustics, Speech and Signal Processing (ICASSP), Singapore, Singapore. DOI: 10.1109/ICASSP43922.2022.9746062
- Florescu, Dorian/Bhandari, Ayush (2022). Unlimited Sampling via Generalized Thresholding. At: 2022 IEEE International Symposium on Information Theory (ISIT), Espoo, Finland. DOI: 10.1109/ISIT50566.2022.9834687
- Shtendel, Gal/Bhandari, Ayush (2022). HDR-TOF: HDR Time-of-Flight Imaging via Modulo Acquisition. At: 2022 IEEE International Conference on Image Processing (ICIP), Bordeaux, France. DOI: 10.1109/ICIP46576.2022.9897552
- Beckmann, Matthias/Bhandari, Ayush (2022). MR. TOMP : Inversion of the Modulo Radon Transform (MRT) via Orthogonal Matching Pursuit (OMP). At: 2022 IEEE International Conference on Image Processing (ICIP), Bordeaux, France. DOI: 10.1109/ICIP46576.2022.9897428
Team
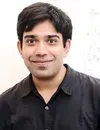
Principal Investigator (Imperial)
Dr. Ayush Bhandari
Lecturer Electrical and Electronic Engineering | Imperial
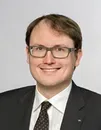
Principal Investigator (TUM)
Prof. Felix Krahmer
Associate Professor for Optimization and Data Analysis
Doctoral Candidate (Imperial)
tba.
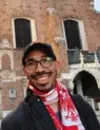